Dario Grana
Professor
Wyoming Excellence Chair
Department of Geology and Geophysics
University of Wyoming
Bayesian Learning Consortium (BLC)
Overview:
The Bayesian Learning Consortium is an industry-sponsored research consortium focusing on advanced quantitative methods for subsurface characterization. The scope of this document is to provide the Executive Director of the School of Energy Resources and the Vice President for Research the necessary information for the approval process. This document includes a description of the project, the research goals, the consortium organization, and the contact information of the director.
Research Goals:
The goal of the consortium is to develop innovative quantitative methods to improve the characterization of subsurface reservoirs for hydrocarbon exploration and carbon dioxide sequestration and quantify the uncertainty in the numerical models.
Research Description:
The estimation of petrophysical properties from seismic data can be formulated as a probabilistic inverse problem in which the goal is to predict the posterior probability distribution of the petrophysical properties at each location in which seismic data are available. Bayesian inversion methods for the estimation of reservoir properties from seismic attributes and for the prediction of elastic attributes from seismic data have been presented in literature. A two-step (sequential) approach combining these two methods does not allow to correctly quantify the uncertainty in the solutions. Furthermore, these methods generally require a geophysical forward model (seismic modeling or rock physics) for the estimation of the likelihood function; however, such models might not be unique in the entire reservoir or can be facies dependent. In our approach, we propose a set of mathematical methods where facies and reservoir properties are jointly estimated from seismic data with different options including full rock physics model, machine learning approaches, hidden Markov chain models. In particular, the challenge of the non-uniqueness of the rock physics relations will be faced by generating a large training dataset and extracting the model-data relation using statistical methods such as kernel density estimation or machine learning algorithms such as convolutional neural network. The natural ordering of facies is preserved by using generating a large number of realizations using the Markov model approach. Rock physics models can then be defined in each facies. The uncertainty due to the non-uniqueness of the solution is quantified using stochastic sampling methods based on Markov chain Monte Carlo algorithms.
Organization:
The consortium will be led by Prof. Dario Grana and will initially include the members of the Seismic Reservoir Characterization research group (currently 3 PhD students, 1 MS student, and 1 postdoc). Prof. Dario Grana will be the contact for all the companies. The consortium will initially include a limited number of companies with common research interest and goals and will share the same dataset for tests and validation. The initial duration of the project is 5 years. Research goals and directions will be reviewed and updated after year 3.
Deliverables:
-
Prototype software
-
Support for in-house applications
-
Students know-how
-
Annual reports
-
SEG/EAGE extended abstract co-authorship
Software:
The codes are available to the consortium members through this passwrod protected link.
Basic MATLAB package:
Administrative Information:
Director: Dario Grana
Department: School of Energy Resources
Current Sponsors:
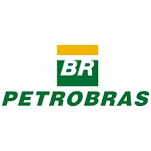

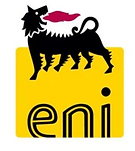